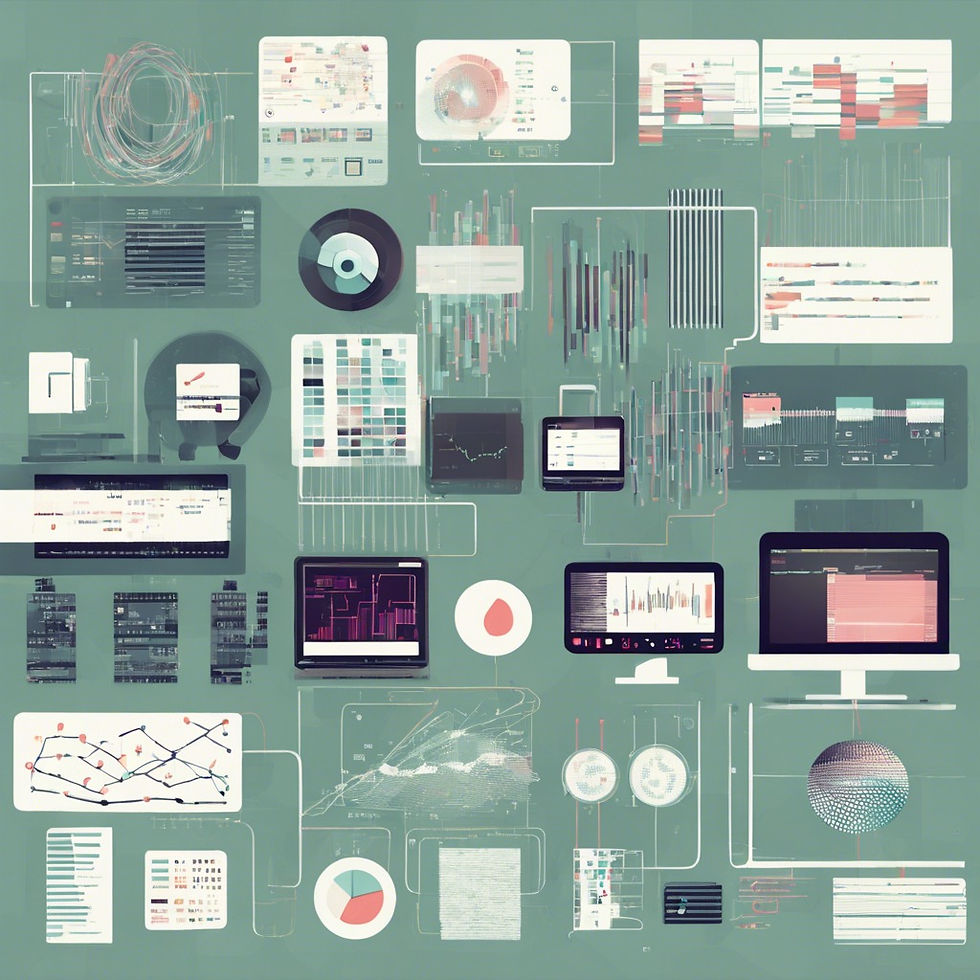
Synthetic data, generated artificially by computers or algorithms using real-world data sets as a basis, is experiencing a surge in demand across various fields as researchers acknowledge its potential. This data type is renowned for its capacity to train machine learning models effectively, mitigate biases within data sets, and address ethical and privacy concerns inherent in using genuine data. Exploring synthetic data entails delving into its creation process, its applications across industries, and the methods for generating bespoke synthetic data sets tailored to specific needs.
Understanding Synthetic Data
Synthetic data refers to artificially generated data that does not contain real values. For instance, if you collect height measurements from five individuals, that's real data. Conversely, creating height measurements for five imaginary individuals constitutes synthetic data.
A handful of algorithms often generate synthetic data to simulate real-world data structures. These algorithms mimic missing values in larger datasets by inferring the data's structure and distribution, then creating plausible false data points. Synthetic data sets find applications in software development, refining machine learning models, and safeguarding sensitive information.
Recent research suggests that models trained on synthetic data tend to outperform those trained on real data. A study by MIT-IBM Watson Lab showed that video processing algorithms achieved better object recognition when trained on synthetic data derived from publicly available datasets compared to actual footage.
Strengths of synthetic data
Synthetic data presents several advantages, such as enabling researchers to analyze data and develop algorithms while safeguarding participants' privacy and security. It serves educational purposes by aiding learners and professionals in comprehending various data structures and types, facilitating the development of practical coding and methodological skills.
Synthetic data offers the flexibility to generate data on demand, devoid of regulatory, security, or ethical constraints. Researchers can tailor synthetic data to include specific features, such as "edge cases," which are rare events crucial for effective training models. By designing synthetic data, researchers can also mitigate biases inherent in real data, adjusting the data structure accordingly.
Weakness of synthetic data
Although synthetic data offers numerous advantages, it also carries potential drawbacks. Poorly generated synthetic data may contain biases, lack diversity, and fail to meet the quality standards for effective model training and real-world data representation.
Employing generative adversarial networks (GAN) to produce data can pose challenges in ensuring accurate data distribution. While researchers might opt for probabilistic statistical models to generate evenly distributed synthetic data sets, there's a risk that these models may not faithfully represent the actual data.
Ensuring the quality and accuracy of synthetic data sets is paramount during their creation. Inaccurate data can lead to unreliable model performance. A useful guideline is that synthetic data derived from viable and comprehensive real-world data tends to be higher quality.
Real-world examples of synthetic data.
Synthetic data has demonstrated effectiveness across various professional domains, offering valuable applications tailored to specific fields. Depending on your expertise, you can explore existing examples of synthetic data utilization or related applications relevant to your area. Recent instances of synthetic data application in diverse industries include:
Medical Imaging
Healthcare professionals can utilize synthetic X-rays to train diagnostic algorithms, bypassing the complexities associated with real-world data handling.
Anthropological Studies
Anthropologists can employ synthetic data to estimate indigenous population sizes and distributions, respecting privacy and addressing limitations due to missing information.
Natural Language Processing
Experts in NLP algorithms can leverage synthetic data for chatbot training, enhancing their ability to handle diverse user interactions through simulated conversations.
Safety Monitoring
Safety management professionals can use synthetic data to train surveillance algorithms for detecting and preventing accidents in high-risk environments like construction sites.
Home Automation
In the realm of home automation, synthetic data can aid in programming robots to interpret and respond to human movements, enhancing user experience and efficiency.
Autonomous Vehicles
Robotics specialists can use synthetic data to enhance the safety features of autonomous vehicles, incorporating scenarios involving unusual pedestrian behavior and unexpected driving situations.
Bias Testing
Researchers can employ synthetically generated data sets to evaluate biases in existing algorithms, refining methodologies to mitigate inherent biases and enhance fairness
How to create synthetic data
When generating synthetic data, you have two main approaches to consider: process-driven generation and data-driven generation.
Your choice between these methods should be guided by the specific purpose and requirements of your dataset.
Process-driven
Involve creating synthetic data using mathematical models that represent an underlying physical process. This approach is suitable when the process is well-understood, such as in fields like physics and engineering. It's also effective for probabilistic modeling, including risk assessment and event simulation.
Data-driven
Utilize generative models based on observed data. These models are often developed through imputations using statistical models or probability distribution methods that analyze the entire data distribution to create a simulated version of it.
Want to learn more about synthetic data? Check out our bootcamps.
Comentaris